10 minute read · February 1, 2024
ZeroETL: Where Virtualization and Lakehouse Patterns Unite

· Senior Tech Evangelist, Dremio
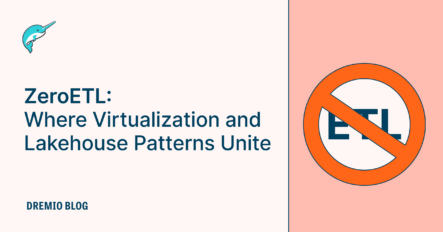
Organizations continually strive to harness the full potential of their data. The traditional approach involves moving data from various sources into a data lake and then into a data warehouse. This process is facilitated by layers of extract, transform, load (ETL) pipelines. While ETL has been a cornerstone of data management strategies, it presents several challenges that impede the efficiency and effectiveness of data-driven decision-making.
The Burden of ETL Pipelines
ETL pipelines are complex constructs. Each pipeline, designed to move and transform data from one stage to the next, requires significant development, testing, deployment, and maintenance efforts. This complexity doesn’t just consume valuable time and resources; it also introduces a high probability of errors.
The primary goal of data management is to provide timely insights that inform business decisions. However, the traditional ETL-based approach often leads to delays. As data moves through multiple processing layers, the time to insight increases. This delay can be costly, especially in a fast-paced business environment where timely information is critical for staying competitive.
With each stage of data movement and transformation, there's a risk of data inconsistency. Discrepancies can arise for various reasons, such as differences in data handling between pipelines or errors in transformation logic. These inconsistencies can lead to unreliable data, which, in turn, affects the quality of insights derived from it.
The development and maintenance of ETL pipelines are not just resource-intensive in terms of manpower. They also incur significant costs in terms of infrastructure and tools required to manage and run these processes. As the data volume grows and the need for more complex transformations increases, these costs can escalate, impacting the overall budget for data management.
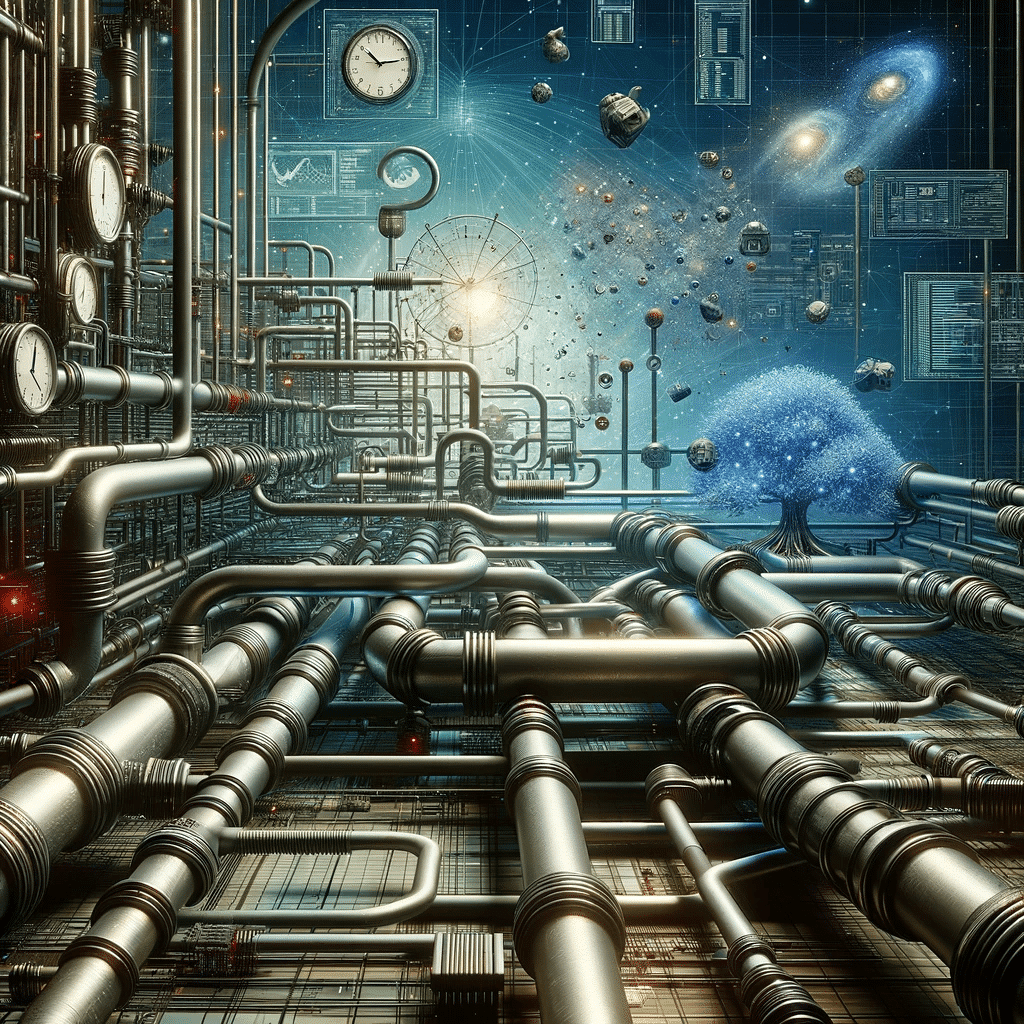
ZeroETL and Low-ETL Patterns
To address these challenges, a paradigm shift is required in handling data. This is where ZeroETL or low-ETL patterns come into play. By leveraging modern architectures such as data lakehouse and data virtualization, organizations can significantly reduce the need for complex ETL processes. This approach not only streamlines data management but also enhances the speed and reliability of insights, all while keeping costs in check.
ZeroETL, or minimal ETL processes, are at the forefront of this transformation, facilitated by two key architectural paradigms: data virtualization and the data lakehouse.
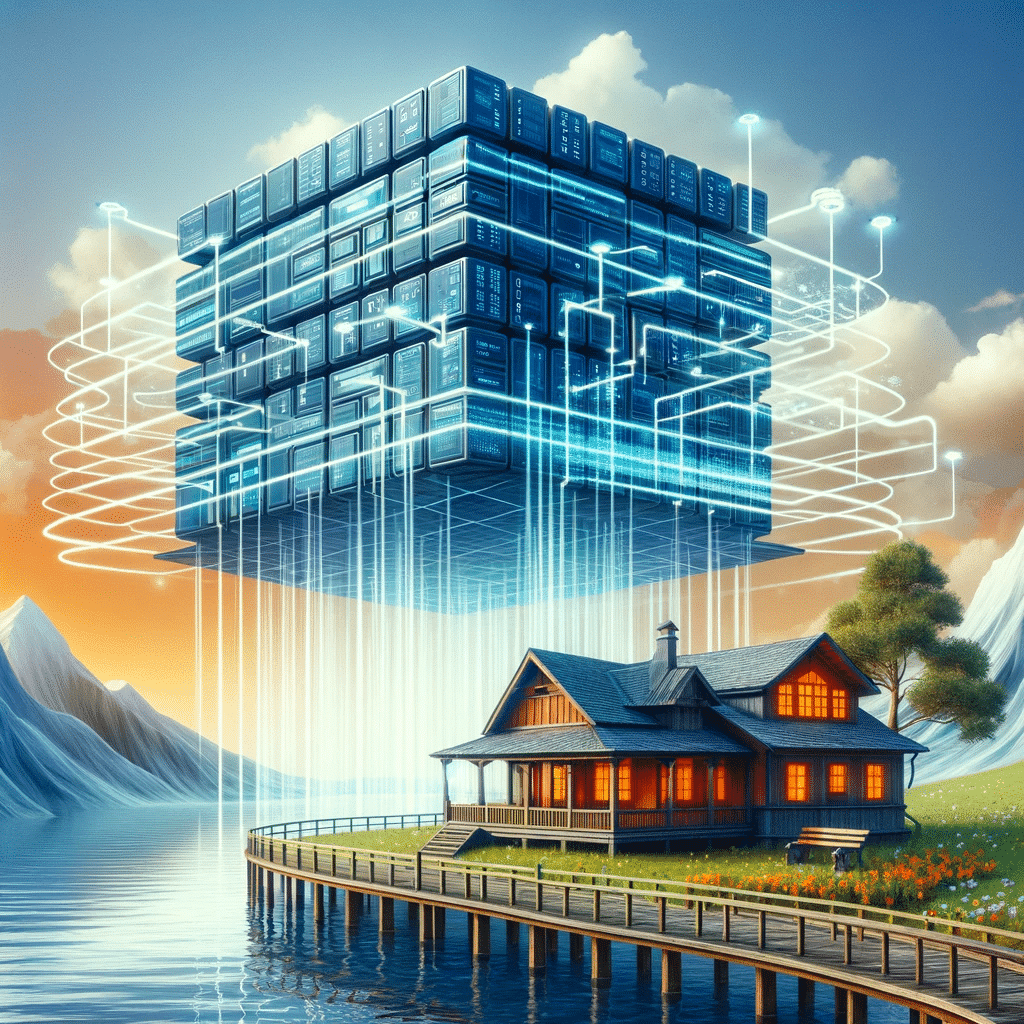
Data Virtualization
Data virtualization allows organizations to access and manage their data without moving it from its original location. This approach creates a single access point to various data sources, enabling real-time or near-real-time data querying and analysis. By eliminating the need for multiple ETL processes to move data, data virtualization significantly reduces the time and resources typically involved in data preparation and integration.
Key benefits of data virtualization:
- Reduced data movement: Direct connection to source data minimizes the need for data movement, thereby reducing the risks associated with data transfer, such as latency and data inconsistency.
- Agility in data access: This enables faster and more flexible access to data, empowering businesses to react swiftly to changing market trends and internal demands.
- Simplified architecture: By removing numerous ETL layers, the architecture becomes less complex, making it easier to manage and scale.
Data Lakehouse
The data lakehouse architecture combines the flexibility and scalability of a data lake with the management features and structured data capabilities of a traditional data warehouse. In a low-ETL data lakehouse, raw data is stored as Apache Iceberg. This approach reduces data movements to a single ingestion process, significantly simplifying the data pipeline.
Advantages of a data lakehouse:
- Single source of truth: Having all data in one place ensures consistency and reliability, providing a comprehensive view of the business.
- Scalability and flexibility: The architecture is scalable to handle large volumes of data and flexible enough to support various data types and sources.
- Cost-effective storage: Utilizing a data lake for storage is often more cost-effective compared to traditional data warehousing solutions.
The Synergy of Virtualization and Lakehouse
The combination of data virtualization and the data lakehouse architecture presents a potent solution for modern data management. By leveraging these approaches, organizations can enjoy the benefits of minimal data movement, reduced pipeline complexity, and faster time to insight, all while maintaining high data quality and consistency.
As a lakehouse platform, Dremio embraces and enhances the principles of data virtualization and data lakehouse architecture. It offers features that streamline data access and analysis, empowering organizations to practice ZeroETL or low-ETL patterns effectively.
Dremio's platform is designed to connect seamlessly with various data sources, enabling direct access to data without needing to move or duplicate it. Dremio also provides complete data lakehouse functionality around Apache Iceberg tables including DDL, DML, and the ability to automate maintenance and cleanup of those tables.
Key features of Dremio:
- Logical data views: Dremio allows the creation of logical views of data. These views act as virtual layers over the physical data, enabling users to model and transform data without physically altering the underlying datasets. This feature significantly reduces the need for traditional ETL processes, as transformations are handled virtually.
- Raw and aggregate reflections: Dremio introduces the concept of reflections, which are optimized data representations. Raw reflections provide a more efficient way to handle detailed queries, while aggregate reflections are tailored for summary and aggregated queries. These reflections remove the need for additional data movement and transformation, thus reducing the workload on data
- Ease of use and maintenance: The platform's user-friendly interface and automated management features make it easy for users to handle data without deep technical expertise. This ease of use extends to the maintenance of the system, as Dremio automates many of the tasks traditionally associated with ETL processes.
The Impact of Dremio in ZeroETL Practices
By adopting Dremio, organizations can significantly streamline their data management processes. The reduced reliance on ETL translates to faster time to insights, lower costs, and improved data consistency. Dremio's approach aligns with the modern needs of businesses, where agility and efficiency in data management are paramount.
- Reduced complexity: Simplifies the data architecture by minimizing redundant data movements and transformations.
- Enhanced data quality: Offers better control over data, leading to improved consistency and reliability.
- Cost-efficiency: Lowers the overall cost of data management by reducing the need for extensive infrastructure and resources traditionally required for ETL processes.
Conclusion
Dremio's Lakehouse platform represents a significant step forward in the evolution of data management. By leveraging data virtualization and lakehouse architecture, it offers a viable solution to the limitations of traditional ETL-based approaches. Organizations embracing Dremio can expect an improvement in their data management capabilities and a strategic advantage in the fast-paced world of data-driven decision-making.
We have explored the challenges of traditional ETL, the benefits of ZeroETL and low-ETL patterns, and how Dremio facilitates these modern data management strategies. As we move forward, it's clear that the future of data management lies in efficiency, flexibility, and the intelligent use of technology like Dremio to swiftly turn data into valuable insights.
Create a Prototype Data Lakehouse on Your Laptop with this Tutorial