11 minute read · February 1, 2024
What Is a Data Lakehouse Platform?

· Senior Tech Evangelist, Dremio
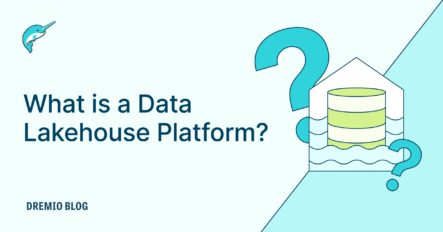
The concept of a data lakehouse is gaining significant traction. This innovative approach represents a paradigm shift from the traditional data warehouses many businesses have relied upon for years. At its core, a data lakehouse is a hybrid that combines the flexibility and scalability of a data lake with the structured organization and management features of a data warehouse. To wrangle all the components of data lakehouse into a single cohesive and easy-to-use data platform, we need a product like Dremio, the data lakehouse platform. In this article, we'll delve into what a data lakehouse platform is, how it makes data lakehouses easier to adopt and use, and why Dremio is becoming a go-to choice for businesses looking to harness the power of their data in the most efficient way possible.
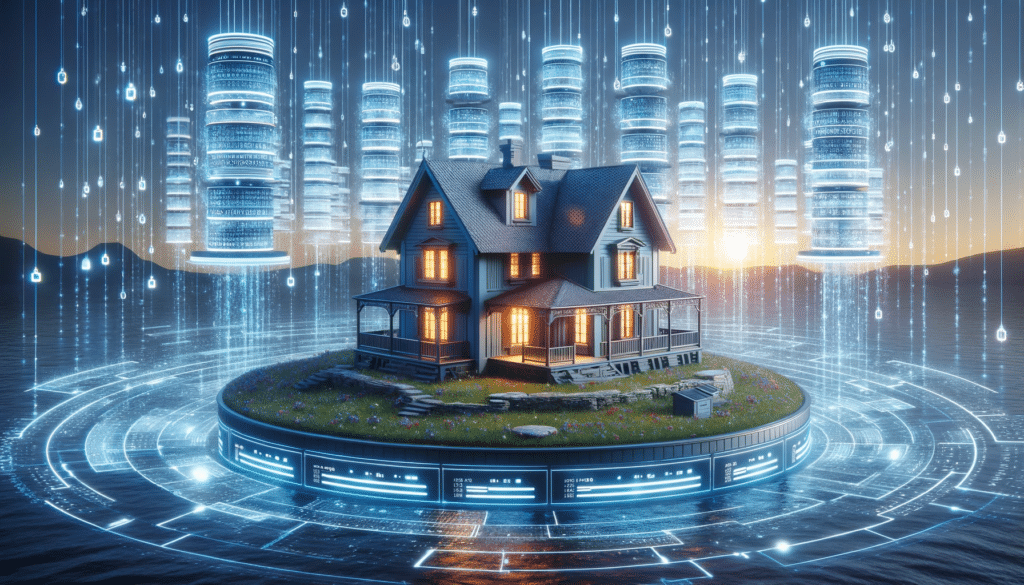
Understanding the Data Lakehouse
To fully appreciate the significance of a data lakehouse platform, it's essential first to understand what a data lakehouse is. A data lakehouse is a modern architecture that allows storing, managing, and analyzing vast amounts of structured and unstructured data through decoupled components that re-create the functionality of data warehouses on your data lake. Lakehouses break down the barriers of traditional data warehouses, which often operate in silos, and offer a more integrated, flexible solution.
Traditional data warehouses are like walled gardens — highly structured but limited in scalability and agility. They are designed for specific purposes and often require data to be processed and formatted in a particular way before it can be used. On the other hand, data lakes are vast pools of raw data stored in their native format, offering immense scalability but often lacking in governance and organization.
The data lakehouse model merges the best of both worlds. It allows for storing massive amounts of raw data (like a data lake) while providing the structure and tools for efficient data processing and analysis (like a data warehouse). This deconstructed approach means that each component — storage, data files, table format, catalog, and compute — is implemented as a decoupled, open bundle, ensuring greater flexibility and scalability.
The Role of Dremio, the Data Lakehouse Platform
Dremio addresses the challenge of integrating the disparate components of a data lakehouse into a cohesive, user-friendly system. Dremio stands out by making it easier for businesses to work with existing data systems, their preferred data lake storage (cloud/on-prem, object storage/HDFS) and with table formats like Apache Iceberg in a more integrated manner. This integration is crucial for companies looking to leverage their data without being bogged down by the complexities of managing the different components of the data lakehouse and making maintenance overwhelming.
One of the critical challenges in creating a data lakehouse is achieving the seamless feel of a traditional data warehouse while maintaining the openness and flexibility of a data lake. Dremio effectively bridges this gap. It provides a platform that supports the diverse needs of modern data storage and analysis and ensures that these components work together harmoniously, mimicking the form factor of a traditional data warehouse but with much greater flexibility and scalability.
Data Integration and Virtualization with Dremio
Data integration is a critical aspect of any data management system, and Dremio excels in this area with its robust data virtualization features. The platform allows seamless data integration from various sources, such as metastores, databases, and other data warehouses. This capability is particularly valuable in today's data-driven world, where businesses often need to pull insights from a diverse range of data repositories, especially when building data products in the creation of a data mesh.
Dremio's data virtualization feature simplifies supplementing and enriching lakehouse data. Instead of moving or copying data into a central repository, Dremio allows users to access and query data where it resides. This approach not only reduces the time and resources spent on data migration but also minimizes the risk of data silos and makes it easier to access different third-party data and use it alongside your data lakehouse tables. Dremio empowers organizations to make more informed decisions based on a comprehensive data picture by providing a unified view of all data, regardless of its location.
Performance and Acceleration Features of Dremio
One of the standout features of Dremio is its Apache Arrow based high-performance query engine, designed to handle complex queries across large datasets with speed and efficiency. This engine is a game-changer for businesses that require real-time analytics and insights, as it drastically reduces the time to insight.
Furthermore, Dremio incorporates innovative acceleration features that enhance its performance. These include reflections, which are Dremio's way of optimizing queries by creating more efficient data representations, and the columnar cloud cache, which improves query performance by caching frequently accessed data. These features ensure that Dremio can handle the demanding workloads of modern data analytics, providing faster and more efficient query performance than traditional data warehouses or lakes.
Semantic Layer and Self-Service Data Governance
Beyond its integration and performance capabilities, Dremio offers a semantic layer that significantly enhances data organization, curation, and governance. This layer allows users to define and manage how data is presented and accessed, ensuring consistency and clarity across the organization. It bridges raw data and business intelligence tools, enabling users to work with data more intuitively and business-friendly.
The semantic layer in Dremio is not just about ease of access; it also plays a crucial role in data governance. It provides a structured way to manage data security, compliance, and quality, ensuring that only authorized users have access to sensitive information. This feature is crucial for businesses operating in regulated industries or dealing with confidential data.
Moreover, Dremio’s approach to data governance empowers users with self-service capabilities. It enables them to access and analyze data without constant reliance on IT teams, fostering a culture of data democratization. This self-service model significantly shifted from the traditional gatekeeper approach, where IT departments had sole control over data access and usage. With Dremio, users across different departments can leverage data insights to drive decision-making, fostering a more data-driven organizational culture.
Lakehouse Management Features in Dremio
Central to Dremio's appeal is its suite of integrated lakehouse management features, designed to streamline and enhance the data management process. One of the standout features is Dremio’s Nessie-based lakehouse data catalog, equipped with Git-like semantics. This catalog provides a comprehensive view of all your lakehouse tables and views, making it easier for users to discover and manage data across the organization. The Git-like semantics bring version control capabilities to data management, enabling better tracking of changes and collaboration among teams.
Automated table optimization is another critical feature of Dremio's platform. This functionality optimizes how your lakehouse tables are stored and cleans up expired data, ensuring efficient data handling and query performance. This feature is particularly important in environments with large and evolving datasets, where manual optimization can be resource-intensive and prone to errors.
Furthermore, Dremio's user interface is vital in enhancing visibility and control. The intuitive UI allows users to easily monitor and manage activity within the data catalog, providing insights into usage that is occurring across the catalog. This visibility is vital for maintaining optimal performance and ensuring the data lakehouse operates smoothly.
Adopting a Data Lakehouse Platform
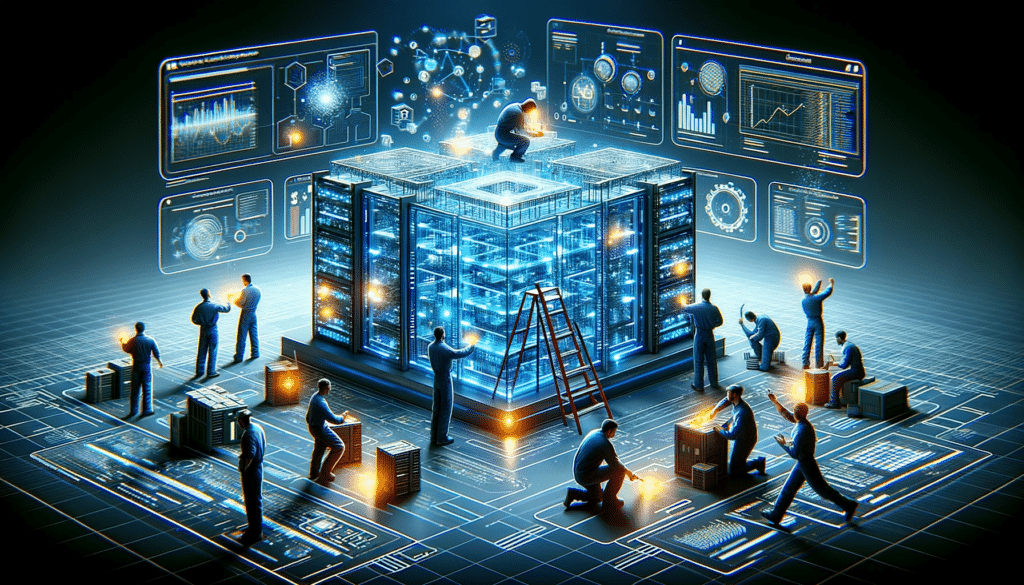
Adopting a data lakehouse architecture with a platform like Dremio offers organizations unparalleled flexibility and accessibility in their data management practices. Dremio's architecture is designed to be open and compatible with various storage solutions, table formats, databases, and data warehouse systems. This openness allows businesses to select the components that best fit their needs and integrate them into a cohesive and efficient data management system.
Dremio also facilitates a gradual and flexible adoption process. Organizations can start small, using only the necessary components, and scale up as their requirements grow. This approach reduces the initial investment and complexity, making it easier for businesses to transition to a data lakehouse architecture at their own pace.
The data lakehouse model combines the best aspects of data lakes and warehouses. Data lakehouses offer a flexible, scalable, and efficient solution for managing and analyzing vast data. Dremio, with its robust integration, performance, governance, and management features, stands out as a leading platform in this space. It simplifies complex data management tasks and democratizes data access, enabling organizations to leverage their data assets more effectively.
As businesses navigate big data challenges, adopting data lakehouse architectures will likely become increasingly prevalent. Data lakehouse platforms like Dremio are at the forefront of this shift, offering a glimpse into the future of data management. This future is more open, integrated, and accessible than ever before.
Create a Prototype Data Lakehouse on Your Laptop with this Tutorial
Sign up for AI Ready Data content