4 minute read · July 25, 2019
Why there isn’t an Apache Arrow article in Wikipedia
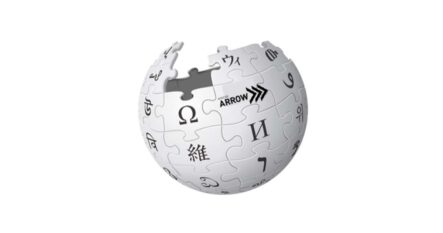
We love Apache Arrow. And it has millions of downloads per month. Seems like it should have a Wikipedia article, much like many other popular open source projects, no? So we took it upon ourselves to contribute one.
All we have to do is abide by their guidelines, namely: “The topic should be notable and be covered in detail in good references from independent sources.” Simple, right? The next step is that an editor or three would take a look at my article, I would make revisions based on their comments, and it would get approved. Well…that’s not quite what happened.
In fact, the article has been submitted 4 times, not just by me but also by others, and declined each and every time.
Understanding Arrow
Arrow is designed to serve as a shared foundation for SQL execution engines, data analysis systems, storage systems, and more – think Pandas, Spark, Parquet, etc. Engineers across the community are working together to establish Arrow as a standard for columnar in-memory processing. In fact, developers from over 13 major open source projects have been involved in the project so far.
Apache Arrow has broad community adoption, with close to 3 million downloads per month. It’s used as much as other open source projects and is a well-known project in the Apache community. In fact, Apache Arrow went straight to top-level status at the Apache Software Foundation instead of starting out in incubation. (And of course, Dremio is a major contributor to Apache Arrow, but we don’t own it and we don’t profit from it. That’s an important distinction.)
Why is it so difficult to get open source project articles accepted on Wikipedia?
I first submitted our Apache Arrow article to Wikipedia back in 2018. Editors said that my page was “not adequately supported by reliable sources,” the subject “doesn’t qualify for a Wikipedia article,” and “reads more like an advertisement.” We made adjustments to the article based on their comments, and then I reached out for feedback on the draft in the Wikipedia talk page. Great news! The reviewers thought that it would be accepted. It was not—the article has now been declined four times. What’s puzzling is that there are many other open source Wikipedia articles, such as Apache Sqoop and Apache Drill, that have made it through the editing gauntlet.
I think the issue here is that my particular Wikipedia article reviewers are not well-versed in nature of open source projects, particularly Apache Arrow, which is subtle since it’s not a standalone piece of software. And I must reiterate that Dremio does not profit from any way from Apache Arrow. It’s not a product; it’s a framework.
So given my experience, I’m wondering if Wikipedia can continue to be considered a reliable source of information for technical folks who want to learn more about the vast system of Apache open source software projects.
Improving the system
It’s interesting to note that Wikipedia has been experiencing growing pains over the past several years. The number of accepted articles continues to grow rapidly, while the number of Wikipedians (editors) is in decline. In fact, the number of editors has shrunk by more than a third since 2007 and is still shrinking. How can this “collaborative encyclopedia” continue to be a credible resource if non-technical Wikipedians are editing technical articles?
It’s well known that visitors come to Wikipedia to acquire knowledge, and others come to share knowledge. Since Wikipedia doesn’t have an organized “staff” that can be assigned particular content areas, I implore Wikipedia to modify their policies and guidelines so that non-technical Wikipedians are actively discouraged from editing technical articles. After all, accuracy builds credibility.
Do you have any suggestions? I’d love to hear them. And if you want to check out the current state of the Apache Arrow article on Wikipedia, you’ll find it here.