10 minute read · January 15, 2025
Key Takeaways from the 2025 State of the Data Lakehouse Report: Navigating the AI Landscape

· Technical Evangelist, Dremio
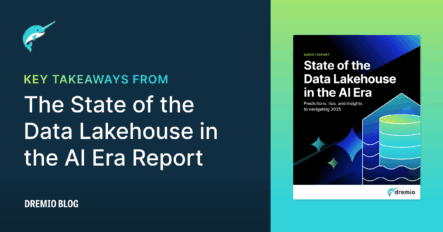
Introduction
The convergence of data and artificial intelligence (AI) continues to reshape the business landscape at an accelerated pace. Organizations are under increasing pressure to derive actionable insights from their data and leverage AI to drive competitive advantage. The 2025 State of the Data Lakehouse in the AI Era Report provides a comprehensive overview of the current trends, challenges, and opportunities defining the future of data and analytics. This analysis delves into the report's key findings, offering strategic insights for organizations seeking to optimize their data ecosystems in the modern, AI-driven world.
AI-Ready Data
A paramount insight from this year's report is the escalating significance of AI-ready data. As businesses aggressively integrate AI and machine learning (ML) into core operations, the quality, unity, and accessibility of data for AI workflows have transitioned from a desirable attribute to a strategic imperative.
Key Statistics:
- 85% of organizations are currently leveraging data lakehouses to underpin AI model development.
- 11% are actively planning to adopt this approach in the near future.
- 36% cite data governance and security as significant impediments to adoption.
- 33% highlight the cost and complexity associated with data preparation as major challenges.
Strategic Implications:
Organizations that prioritize the development of AI-ready data ecosystems—emphasizing accessibility, robust governance, and unwavering data quality—are better positioned to:
- Accelerate innovation cycles.
- Enhance decision-making through data-driven insights.
- Optimize operational efficiency and reduce costs.
- Achieve significant competitive advantages.
Open Standards are Reinforced
The hybrid lakehouse model, with its commitment to open standards, continues to gain traction. This approach empowers businesses with the agility and flexibility necessary to adapt to evolving technological landscapes and business requirements.
Key Statistics:
- Open table formats and metadata solutions, such as Apache Iceberg and Polaris, are gaining widespread adoption, fostering interoperability and healthy competition among vendors.
Strategic Implications:
By embracing the philosophy of "your storage, your data, your metadata," organizations can:
- Mitigate the risks of vendor lock-in.
- Ensure the long-term viability and adaptability of their data architectures.
- Unlock the full potential of their data ecosystems by fostering a culture of innovation and collaboration.
Balancing Centralization with Decentralization
The report reveals a nuanced shift in strategic priorities as organizations refine their data strategies for the AI era.
Key Observations:
- Data Mesh Strategies: While full implementations of data mesh architectures experienced a slight decrease (6%), partial adoption remains strong. This suggests a move towards a more pragmatic, phased approach to decentralization. Priorities are shifting toward improved data access and democratized data architectures, reflecting a growing need for agility and scalability.
- Consolidation of Analytics: A compelling 90% of IT decision-makers are actively pursuing the consolidation of analytics data into a single, unified location—a 4% increase from the previous year. This underscores the importance of streamlined, cohesive data strategies that enhance analytical capabilities.
Strategic Implications:
These trends indicate a growing recognition of the need to strike a delicate balance between:
- Centralized governance and oversight: Ensuring data quality, security, and compliance.
- Decentralized access and autonomy: Empowering business units with self-service capabilities and fostering data democratization.
This balanced approach is crucial for achieving both scalability and agility while maintaining robust data governance.
AI-Ready Data Products
Data products, particularly those designed with AI readiness in mind, are revolutionizing how businesses manage, govern, and leverage their data assets.
Key Statistics:
- 65% of organizations have established formalized processes for the creation and management of data products.
- 98% demonstrate at least a basic familiarity with the concept of data products.
Strategic Implications:
- Autonomous capabilities, exemplified by solutions like Reflections, are empowering organizations to optimize performance, minimize latency, and ensure that data products deliver tangible, measurable business value.
- The focus is shifting towards treating data as a product, enabling faster innovation and more efficient data utilization across the organization.
Barriers to Self-Service Analytics: Empowering the Data Citizen
While 80% of organizations aspire to democratize data access through self-service initiatives, significant obstacles persist.
Key Challenges:
- Security and Governance Concerns: Cited by 42% of respondents.
- Tool Complexity: A barrier for 24%.
- Lack of Adequate Training and Support: Identified by 19%.
Strategic Implications:
Addressing these challenges is paramount to unlocking the full potential of self-service analytics and empowering a broader range of users to:
- Work more efficiently and independently.
- Derive insights directly from data without relying solely on specialized data teams.
- Contribute to a data-driven culture across the organization.
Operational Pain Points
Data and analytics professionals express a mix of satisfaction and frustration regarding their roles.
Key Insights:
- Most Enjoyable Tasks: Streamlining workflows (30%) and discovering meaningful patterns in data (20%) are considered the most rewarding aspects of their work.
- Least Enjoyable Tasks: Manual, repetitive processes (28%) and cleaning raw data (19%) remain significant pain points, highlighting the need for automation and intelligent data management solutions.
Strategic Implications:
- Investing in tools and processes that automate manual tasks and streamline data preparation will not only enhance productivity but also significantly improve job satisfaction among data professionals.
- Automation frees up data teams to focus on higher-value activities, such as strategic analysis and innovation.
Conclusion
The 2025 State of the Data Lakehouse Report underscores a pivotal juncture for organizations navigating the evolving data landscape. To thrive in this AI-driven era, businesses must:
- Prioritize AI-Ready Data: Invest in unified, high-quality data infrastructure that serves as the foundation for advanced analytics and AI initiatives.
- Embrace Open Standards: Leverage open technologies like Apache Iceberg and Polaris to ensure flexibility, interoperability, and future-proof data architectures.
- Streamline Operations: Implement automation to address inefficiencies in data workflows, reduce overprocessing, and empower teams with robust self-service capabilities.
- Foster a Data-Driven Culture: Promote data literacy and provide the necessary tools and training to enable data democratization across the organization.
The hybrid lakehouse architecture remains a central enabler for achieving these objectives, providing a powerful framework that balances cost efficiency, scalability, and innovation. By aligning with these strategic imperatives, organizations can position themselves for sustained success in an increasingly complex and competitive, AI-powered world.