35 minute read · September 24, 2024
How Dremio brings together Data Unification and Decentralization for Ease-of-Use and Performance in Analytics

· Senior Tech Evangelist, Dremio
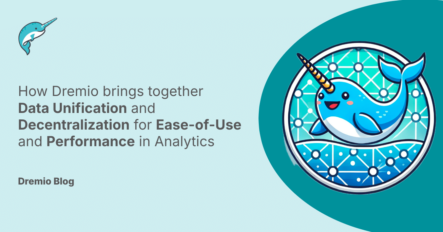
The scale, speed, and variety of data are growing exponentially. Organizations are inundated with vast amounts of information from an ever-increasing number of sources, ranging from traditional databases to cloud-based systems and real-time data streams. This data deluge presents significant challenges for traditional data architectures, which often rely on extensive data pipelines and centralized storage solutions like data lakes and warehouses. These conventional systems struggle to keep pace, becoming too slow, rigid, and costly to meet modern business needs.
The crux of the problem lies in data silos and the inefficiencies they breed. Isolated pockets of data scattered across different departments or systems hinder collaboration, slow down decision-making, and lead to redundant efforts. Businesses find obtaining a unified view of their data increasingly difficult, resulting in missed opportunities and impaired agility in a competitive market.
To overcome these challenges, a transformative approach is emerging that combines data unification and decentralization. Data unification aims to provide centralized access to all data, breaking down silos and enabling seamless analytics across the organization. On the other hand, data decentralization empowers individual teams to manage and access data independently, fostering flexibility and faster innovation.
Enter Dremio, a data lakehouse platform uniquely combining data unification and decentralization. Dremio bridges the gap between centralized access and decentralized data management, offering a solution that enhances analytics performance, scalability, and ease-of-use. By leveraging open-source technologies like Apache Arrow, Apache Iceberg, and Project Nessie, Dremio enables organizations to harness the full potential of their data while maintaining the agility needed in today's fast-paced environment.
This article explores how Dremio achieves this balance, delving into the data unification and decentralization concepts, the trends driving these approaches, and the technologies that make it all possible.
Understanding Data Unification and Decentralization
What Is Data Unification?
Data unification refers to consolidating data access within an organization, providing a centralized platform where all data sources are accessible and manageable. The primary goal is to eliminate data silos by integrating disparate data repositories—such as databases, data warehouses, and data lakes—into a single, cohesive environment. This unified access layer allows stakeholders across the organization to retrieve, analyze, and share data seamlessly, regardless of where the data physically resides.
Benefits of data unification include:
- Enhanced Collaboration: Teams can work together more effectively when they have access to the same data sets.
- Improved Decision-Making: A unified view of data ensures that decisions are based on comprehensive and consistent information.
- Increased Efficiency: Reducing the need for data movement and duplication streamlines workflows and reduces costs.
- Simplified Data Governance: Centralized access makes implementing security measures and compliance protocols easier across all data assets.
What Is Data Decentralization?
Data decentralization, in contrast, involves distributing data storage and management across various systems, teams, or locations. Rather than consolidating data into a single repository, decentralization empowers domain-specific teams to own and manage their data assets. Each team is responsible for preparing, curating, and maintaining their data, treating it as a product that serves the needs of their specific domain.
Key aspects of data decentralization include:
- Domain Ownership: Teams closest to the data and its context manage it, ensuring higher data quality and relevance.
- Flexibility and Scalability: Decentralization allows organizations to adapt more quickly to changes by enabling independent scaling of data domains.
- Innovation Enablement: Teams can choose technologies and methodologies that best suit their data needs, fostering innovation.
- Reduced Bottlenecks: Eliminating reliance on a central data team reduces delays and accelerates data delivery.
The Synergy Between the Two
At first glance, data unification and decentralization might seem like opposing strategies. However, when effectively combined, they offer a powerful approach to data management that leverages the strengths of both.
The synergy lies in unifying access to data while decentralizing its ownership and preparation. This means that while data remains distributed across various domains and systems, users can access and analyze it through a centralized interface. This hybrid approach provides several advantages:
- Centralized Access, Decentralized Control: Users benefit from a unified view of data without interfering with the autonomy of domain teams.
- Enhanced Agility: Decentralized teams can make rapid changes and improvements to their data products without impacting the entire system.
- Improved Data Quality: Domain experts manage their data, leading to more accurate and contextually relevant data sets.
- Scalable Governance: Centralized policies and security measures can be enforced across all data assets, simplifying compliance.
By adopting both data unification and decentralization, organizations can overcome the limitations of traditional data architectures. They achieve the flexibility and speed of decentralized data management while maintaining the coherence and accessibility of a unified data platform.
Dremio embodies this combined approach. It provides a unified access layer through its data lakehouse platform, allowing users to query and analyze data from various sources seamlessly. At the same time, it supports decentralization by enabling domain teams to manage and prepare their own data assets using the tools and systems that best suit their needs. This balance results in enhanced performance, ease-of-use, and the ability to derive insights more quickly and efficiently.
Trends Driving Data Decentralization
The shift towards data decentralization in modern data architectures is fueled by several key trends that address the limitations of traditional, centralized systems. These trends aim to enhance flexibility, scalability, and accessibility, allowing organizations to handle the growing complexity and volume of data effectively. The three significant trends driving this transformation are the emergence of data lakehouses, the adoption of data virtualization, and the implementation of data mesh principles.
Data Lakehouse
The data lakehouse architecture is a hybrid model that combines the expansive storage capabilities of data lakes with the analytical power and transactional support of data warehouses. This approach enables organizations to store vast amounts of structured and unstructured data in a single repository while providing the tools necessary for advanced analytics and business intelligence.
Key features of the data lakehouse include:
- Unified Storage and Analytics: By integrating storage and analytics, data lakehouses eliminate the need for separate systems, reducing complexity and cost.
- Open Formats and Standards: Utilizing open-source technologies and formats allows for greater interoperability and flexibility, avoiding vendor lock-in.
- Support for Diverse Workloads: Data lakehouses can handle a variety of data types and processing needs, from batch analytics, real-time streaming, graph analytics and more.
Benefits of the Data Lakehouse:
- Simplified Data Management: A single platform for all data reduces the overhead associated with maintaining multiple systems.
- Cost Efficiency: Lower infrastructure and maintenance costs due to consolidation.
- Enhanced Performance: Improved query performance through optimized data storage and indexing techniques.
Data Virtualization
Data virtualization provides a way to access and integrate data from multiple sources in real-time without the need to move or replicate it. This technology creates a virtual data layer that connects disparate data systems, allowing users to query and analyze data as if it were stored in a single location.
Advantages of Data Virtualization:
- Real-Time Access: Users can retrieve up-to-date data on-demand from various sources.
- Reduced Data Movement: Minimizes the need for ETL (Extract, Transform, Load) processes, saving time and resources.
- Unified View of Data: Offers a single interface for data access, simplifying analytics and reporting.
Impact on Organizations:
- Agility in Decision-Making: Faster access to data leads to quicker insights and responses to market changes.
- Improved Collaboration: Teams can access the same data sources, fostering better communication and cooperation.
- Lower Costs: Reduces infrastructure expenses associated with data storage and movement.
Data Mesh
Data mesh is a decentralized approach to data architecture that assigns ownership of data to specific domain-oriented teams within an organization. Each team is responsible for their "data products," including data quality, governance, and delivery. This model treats data as a product, managed with the same rigor and standards as any customer-facing offering.
Core Principles of Data Mesh:
- Domain Ownership: Data is owned by the teams that know it best, ensuring relevance and accuracy.
- Data as a Product: Emphasizes delivering high-quality, reliable data that meets user needs.
- Self-Service Infrastructure: Provides teams with the tools they need to manage data independently.
- Federated Governance: Balances autonomy with global standards to maintain consistency and compliance.
Benefits of Data Mesh:
- Scalability: Enables organizations to scale their data practices organically as each domain grows.
- Flexibility: Teams can adopt technologies and processes that best suit their specific needs.
- Faster Time-to-Insight: Reduces bottlenecks associated with centralized data teams, accelerating analytics.
How These Trends Intersect
While each trend addresses different challenges, they collectively contribute to a more agile, scalable, and efficient data architecture. The data lakehouse provides the foundational storage and processing capabilities. Data virtualization overlays this with a unified access layer, allowing for seamless querying across disparate sources. Data mesh empowers individual teams to manage their data autonomously within this framework.
Combined Benefits:
- Enhanced Accessibility: Users can access all relevant data through a unified interface, regardless of where it resides.
- Improved Data Quality: Domain teams ensure that data is accurate and contextually appropriate.
- Operational Efficiency: Reduces duplication of efforts and streamlines data workflows.
- Strategic Agility: Organizations can adapt more quickly to changing business needs and technological advancements.
By embracing these trends, companies can overcome the limitations of traditional data architectures, achieving both data unification and decentralization. This synergy allows for centralized access and governance while enabling decentralized data ownership and innovation.
The Open Source Foundation of the Lakehouse
The modern data lakehouse architecture is built upon a foundation of open-source technologies that provide the flexibility, performance, and interoperability required in today's data landscape. Leveraging open-source components ensures that organizations are not locked into proprietary systems, fostering innovation and collaboration across the data community. Three pivotal open-source projects underpinning the lakehouse architecture are Apache Arrow, Apache Iceberg, and Project Nessie/Apache Polaris (incubating).
Apache Arrow
Apache Arrow is a cross-language development platform for in-memory data. It defines a standardized columnar memory format for efficient data processing and interchange between systems. By enabling zero-copy data sharing between different applications, Arrow significantly reduces the overhead associated with data serialization and deserialization.
Key Features of Apache Arrow:
- High Performance: Optimized for analytics workloads with CPU cache-efficient data structures.
- Interoperability: Supports seamless data exchange between different programming languages and systems.
- In-Memory Computing: Facilitates faster data processing by keeping data in memory in a columnar format.
Impact on Data Analytics:
- Speed Improvements: Accelerates query execution and data processing tasks.
- Resource Efficiency: Reduces memory usage and CPU cycles, lowering infrastructure costs.
- Ecosystem Integration: Widely adopted by many data processing frameworks, enhancing compatibility.
Apache Iceberg
Apache Iceberg is an open table format designed for large analytic datasets. It addresses the limitations of traditional metastore-based tables in big data environments, providing features that enable reliable and efficient data management on top of data lakes.
Benefits of Apache Iceberg:
- ACID Transactions: Ensures data consistency with atomic operations for reads and writes.
- Schema Evolution: Allows for changes to table schemas without disrupting ongoing operations.
- Partition Evolution: Supports dynamic partitioning strategies for optimized query performance.
- Time Travel Queries: Enables querying historical data states, facilitating auditing and debugging.
Enhancements to Data Lakes:
- Improved Data Integrity: Reduces the risk of data corruption and inconsistencies.
- Simplified Data Management: Streamlines operations like compaction, cleanup, and snapshot management.
- Optimized Performance: Enhances query speeds through better data organization and indexing.
Project Nessie & Apache Polaris (Incubating)
Nessie and Apache Polaris are Lakehouse catalogs for tracking your Apache Iceberg tables on your data lake. Project Nessie introduces a Git-like experience for data lakes, providing version control and collaboration features for data stored in systems like Apache Iceberg. Apache Polaris introduces the idea of having internal/external catalogs and robust RBAC features.
Core Capabilities of Project Nessie & Apache Polaris:
- Branching and Merging: Nessie Allows users to create branches for development and testing, merging changes when ready.
- Commit History: Nessie & Polaris Maintains a record of all data changes, supporting audit trails and rollbacks.
- Isolation: Nessie Enables isolated data environments for experimentation without affecting production data.
- Collaboration: Nessie Facilitates teamwork by allowing multiple users to work on different aspects of the data simultaneously.
- Catalog Federation: Apache Polaris enables connections to "external catalogs," like connecting to Nessie, to expose all tables in both catalogs from one access point.
- Multi-Catalog: Apache Polaris allows you to create multiple catalogs that each have their own tables and governance rules.
- RBAC: Apache Polaris has a dual-role-based access control. You can specify catalog roles specifying levels of access to objects in specific catalog and then assign those a principal role which can be attached to users.
Advantages for Data Management:
- Data Governance: Enhances control over data modifications, supporting compliance requirements.
- Operational Agility: Speeds up development cycles by enabling safe experimentation.
- Risk Mitigation: Reduces the likelihood of errors impacting production data.
Integration of Open Source Components
The combined use of Apache Arrow, Apache Iceberg, and Project Nessie/Apache Polaris creates a powerful, open foundation for the data lakehouse architecture. Together, they offer:
- Performance and Efficiency: Arrow's in-memory format and Iceberg's optimized storage structures accelerate data processing.
- Robust Data Management: Iceberg's table format and Nessie's version control ensure data reliability and integrity.
- Flexibility and Interoperability: Open standards facilitate integration with various tools and platforms, avoiding vendor lock-in.
Benefits of an Open Source Approach:
- Community Innovation: Continuous improvements driven by a global community of contributors.
- Cost Savings: Eliminates licensing fees associated with proprietary software.
- Transparency: Access to source code allows for greater understanding and customization.
Dremio's Role in Leveraging Open Source Technologies:
Dremio integrates these open-source components to deliver a seamless and efficient data lakehouse platform:
- Apache Arrow: Utilized for high-speed, in-memory query processing, enhancing performance.
- Apache Iceberg: Provides the table format for managing large datasets with transactional consistency.
- Lakehouse Catalog: Dremio offers the ability to connect to Nessie and Apache Polaris to leverage their capabilities within Dremio. The integrated Dremio Catalog takes the best from these open-source projects for a deeply integrated experience.
By building on these technologies, Dremio empowers organizations to create an open lakehouse architecture that is performant, scalable, and adaptable to changing data needs. This integration supports data unification and decentralization, allowing for centralized access to data while enabling domain teams to manage their data assets independently.
Overcoming Data Silos with Dremio
Data silos have long been a significant obstacle for organizations striving to become truly data-driven. These silos arise when data is isolated within specific departments or systems, making it inaccessible to others within the organization. This fragmentation leads to inefficiencies, as teams duplicate efforts or make decisions based on incomplete information. The lack of a holistic view hampers collaboration, slows down innovation, and can result in missed opportunities.
Try this exercise to first hand see how Dremio deals with Data Silos.
The Challenge of Data Silos
Data silos typically emerge due to a combination of organizational structure, diverse technologies, and cultural barriers:
- Organizational Structure: In companies with rigid departmental divisions, each unit may generate and store data independently, leading to isolated data repositories.
- Diverse Technologies: Different departments might use various databases, applications, or storage solutions that are not interoperable, complicating data integration efforts.
- Cultural Barriers: A lack of trust or competition between departments can discourage data sharing, further entrenching silos.
The consequences of data silos are far-reaching:
- Inefficient Operations: Redundant data collection and storage increase costs and resource utilization.
- Impaired Decision-Making: Incomplete data leads to decisions that don't consider the full picture, potentially causing strategic missteps.
- Reduced Agility: The inability to access comprehensive data quickly hampers an organization's responsiveness to market changes or emerging trends.
Dremio's Solution
Dremio addresses the challenges of data silos head-on by providing a platform that unifies disparate data sources into a seamless analytics environment. It achieves this unification through data virtualization and a robust semantic layer, allowing users to access and query data from various sources without the need for data movement or duplication.
Key Features Facilitating Unification:
- Data Virtualization: Dremio connects directly to a wide range of data sources, including traditional relational databases, NoSQL databases, cloud storage systems, and even other data warehouses. This connectivity enables the creation of virtual datasets that can be queried as if they were in a single repository.
- Self-Service Data Access: By democratizing data access, Dremio empowers analysts, data scientists, and business users to retrieve and work with data independently. This self-service model reduces reliance on IT teams and accelerates the analytics process.
- Advanced Query Acceleration: Dremio incorporates technologies like Apache Arrow and Data Reflections to optimize query performance. These features ensure that even complex queries on large datasets return results quickly, enhancing user productivity.
- Unified Semantic Layer: Dremio provides a semantic layer that standardizes data definitions and metrics across the organization. This layer ensures consistency in reporting and analytics, as everyone is working with the same definitions and calculations.
How Dremio Unifies Data Sources
Dremio's approach to unifying data involves creating a single point of access to all data sources, regardless of their location or format. Here's how it works:
- Direct Connectivity: Dremio connects to data sources where they reside, whether on-premises or in the cloud. Supported sources include SQL databases like PostgreSQL and MySQL, NoSQL databases like MongoDB and Elasticsearch, cloud storage like Amazon S3 and Azure Data Lake Storage, and even other data warehouses like Snowflake and Redshift.
- Virtual Datasets: Instead of moving data, Dremio creates virtual datasets that reference the underlying data sources. Users can join, transform, and analyze these virtual datasets using standard SQL queries.
- Data Governance and Security: Dremio integrates with existing security protocols and offers fine-grained access controls. This integration ensures that data remains secure and that users only access data they are authorized to view.
Benefits of Dremio's Unified Approach
By overcoming data silos, Dremio delivers several benefits:
- Enhanced Collaboration: With unified data access, teams across the organization can collaborate more effectively, sharing insights and building upon each other's work.
- Improved Efficiency: Eliminating the need for data duplication reduces storage costs and minimizes the time spent on data preparation and ETL processes.
- Faster Decision-Making: Quick access to comprehensive data enables stakeholders to make informed decisions promptly.
- Reduced IT Burden: The self-service model frees up IT resources, allowing them to focus on strategic initiatives rather than ad-hoc data requests.
The Unified Apache Iceberg Lakehouse Approach
The convergence of data lakehouse architecture and data virtualization represents a significant advancement in how organizations manage and analyze their data. This unified approach leverages the strengths of both models to provide a scalable, high-performance platform that meets the demands of modern data analytics.
Combining Lakehouse and Virtualization
Dremio operationalizes data lakes into full-featured lakehouses using Apache Iceberg, an open table format for huge analytic datasets. This combination offers the flexibility of data lakes with the performance and transactional capabilities of data warehouses.
Key Components:
- Apache Iceberg: Iceberg provides a robust table format that supports features like ACID transactions, schema evolution, and time-travel queries. It transforms the data lake into a structured environment suitable for complex analytics.
- Data Virtualization: Dremio's data virtualization layer allows for seamless access to data across various sources, not just the data lake. This capability means that organizations can enrich their lakehouse data with information from databases, data warehouses, and external sources without physical data movement.
Try this exercise to replicate an end-to-end Iceberg Lakehouse experience with Dremio on your Laptop
Dremio as the Unified Access and Governance Layer
Dremio sits atop this architecture as the unified access and governance layer, providing several critical functions:
- Single Semantic Layer: Dremio offers a centralized semantic layer where business logic, data definitions, and metrics are defined consistently. This layer ensures that all users, regardless of their tool of choice, are working with the same data interpretations.
- Robust Security and Governance: With role-based access controls, column and row-level security, and integration with enterprise authentication systems, Dremio ensures that data governance policies are enforced uniformly across all data assets.
- High-Performance Query Engine: Utilizing Apache Arrow and other acceleration technologies, Dremio delivers fast query performance, even on large and complex datasets.
Key Benefits
- Simplified Data Management:
- Reduced Complexity: By unifying data access and management, organizations can simplify their data architecture, reducing the number of systems and integrations required.
- Ease of Maintenance: Centralized governance and standardized processes make it easier to maintain and update the data platform.
- Enhanced Performance and Scalability:
- Optimized Queries: Dremio's query engine optimizes execution plans and leverages Data Reflections to accelerate query performance.
- Elastic Scalability: The platform can scale horizontally to handle increasing data volumes and user concurrency without sacrificing performance.
- Up-to-Date Data for Analytics and AI:
- Real-Time Access: Data virtualization ensures that users are always working with the most current data, vital for accurate analytics and AI model training.
- Time Travel and Versioning: Features like time-travel queries in Apache Iceberg allow users to access historical data states, supporting use cases like auditing and trend analysis.
Integrating Open Source Technologies
Dremio's adoption of open-source technologies like Apache Iceberg, Apache Arrow, and Project Nessie enhances the platform's capabilities:
- Flexibility: Open standards prevent vendor lock-in and allow organizations to integrate with a broad ecosystem of tools and technologies.
- Community Innovation: Leveraging open-source projects means benefiting from the collective advancements made by a global community of developers.
- Cost Efficiency: Utilizing open-source components can reduce licensing costs associated with proprietary solutions.
Realizing the Unified Apache Iceberg Lakehouse
By implementing this unified approach, organizations can achieve:
- A Modern Data Architecture: One that meets the needs of today's data-intensive applications and analytics workloads.
- Empowered Teams: Domain-specific teams can manage their data products effectively while still contributing to a cohesive organizational data strategy.
- Competitive Advantage: Faster insights and the ability to adapt quickly to changing data landscapes provide a significant edge in the market.
Conclusion
Organizations are seeking innovative solutions to harness its full potential. The exponential growth of data has exposed the limitations of traditional architectures, highlighting issues such as data silos, slow processing speeds, and inflexible systems. These challenges impede collaboration, decision-making, and the ability to respond swiftly to market dynamics.
To navigate this landscape, a transformative approach that combines data unification and decentralization has emerged. Data unification provides centralized access to disparate data sources, breaking down silos and enabling seamless analytics across the organization. Simultaneously, data decentralization empowers domain-specific teams to manage and prepare their own data assets, fostering flexibility, innovation, and faster time-to-insight.
Dremio stands at the forefront of this transformation, uniquely bridging the gap between centralized access and decentralized data management. By integrating cutting-edge trends such as data lakehouse architectures, data virtualization, and data mesh principles, Dremio offers a platform that is both powerful and adaptable. It leverages open-source technologies like Apache Arrow for high-performance in-memory processing, Apache Iceberg for robust data lakehouse capabilities, and Project Nessie for version control and data governance.
Through its unified access layer, Dremio overcomes the persistent issue of data silos, providing users with real-time access to a wide array of data sources without the need for data movement or duplication. Its support for self-service data access democratizes analytics, reducing dependency on IT teams and accelerating decision-making processes. The platform's high-performance query engine and advanced features like Data Reflections ensure that even complex queries on large datasets return results swiftly.
By embracing both data unification and decentralization, organizations can achieve a harmonious balance that leverages the strengths of each approach. Centralized access ensures consistency, security, and ease of governance, while decentralized management allows for agility, domain-specific optimization, and innovation. Dremio's Unified Apache Iceberg Lakehouse embodies this balance, providing a scalable, efficient, and user-friendly platform that meets the diverse needs of modern data-driven enterprises.
Key Takeaways:
- Enhanced Performance and Scalability: Dremio's architecture ensures that organizations can handle growing data volumes and complexity without sacrificing speed or efficiency.
- Simplified Data Management: A unified platform reduces architectural complexity, lowers costs, and streamlines maintenance efforts.
- Empowered Teams: By enabling domain teams to manage their own data products within a governed framework, Dremio fosters innovation and faster insights.
- Future-Proofing the Data Strategy: Leveraging open-source technologies and modern architectural trends positions organizations to adapt to evolving data landscapes.
As businesses continue to navigate the challenges of big data, adopting a platform that brings together data unification and decentralization is no longer a luxury but a necessity. Dremio offers a compelling solution that addresses these needs, providing the tools and capabilities required to unlock the full value of data assets.
Schedule a meeting to discuss your challenges and whether Dremio is the solution.