11 minute read · June 14, 2024
Enhancing your Snowflake Data Warehouse with the Dremio Lakehouse Platform
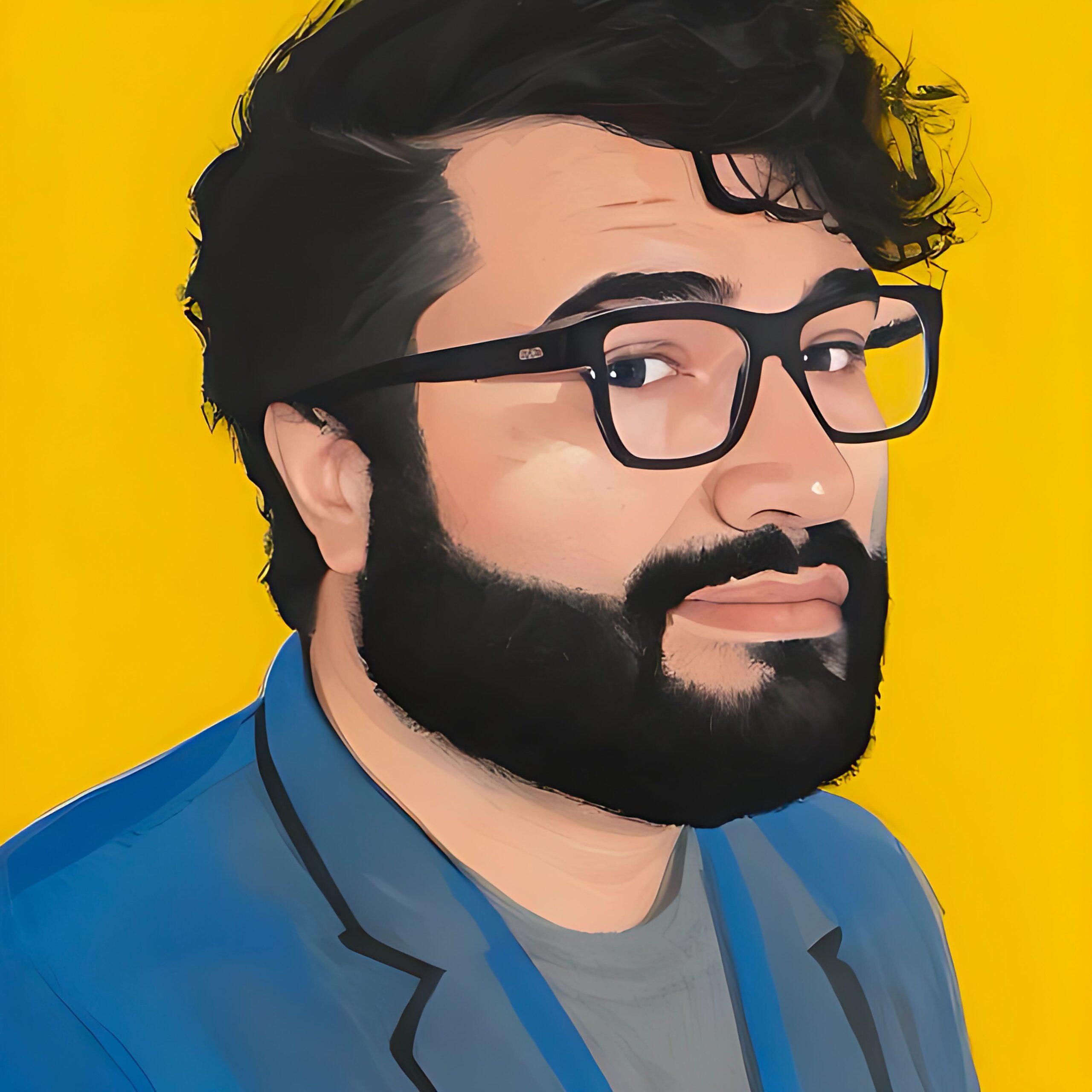
· Senior Tech Evangelist, Dremio
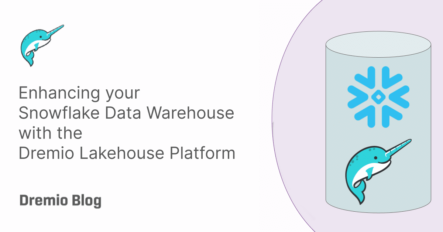
Snowflake is a popular data platform for its scalability, performance, and ease of use. It has revolutionized data warehousing by providing a fully managed service with built-in support for SQL and advanced analytics. Snowflake excels at handling large volumes of data, supporting complex queries, and integrating seamlessly with various data sources and tools.
However, building data platforms is an ongoing evolution. While Snowflake solves many problems exceptionally well, challenges such as data silos, high costs of scaling analytics, dependency on data teams for data access, and proprietary data management formats still persist. These issues can hinder organizations from fully leveraging their data assets to drive decision-making and innovation.
This is where data lakehouse architecture, and specifically the Dremio Lakehouse Platform, comes into play. A data lakehouse combines the best features of data lakes and data warehouses, offering a unified platform that supports structured and unstructured data, facilitates self-service analytics, and optimizes cost and performance. Integrating Dremio with your Snowflake data warehouse can overcome these challenges and achieve a more comprehensive and efficient data platform.
This blog will examine how Dremio complements your Snowflake data warehouse, addressing the key issues and enhancing your data strategy.
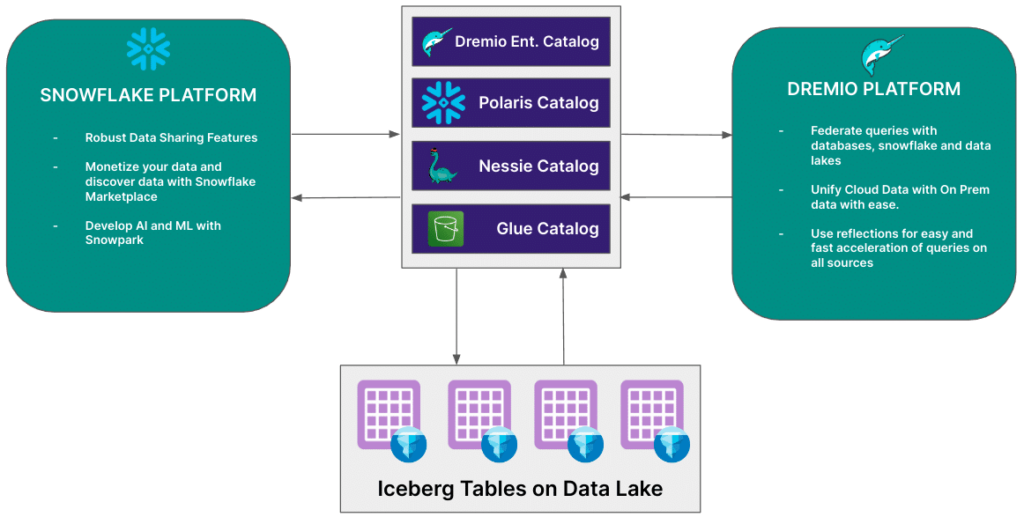
The Challenges of Data Warehouse Platforms
Snowflake provides tremendous value with its easy-to-use platform, data marketplace, and Snowpark feature for creating AI/ML applications. However, there are areas where organizations can encounter friction:
Siloed Data
Data scattered across multiple platforms in the cloud and on-prem, including Snowflake, poses challenges in discovery and cross-silo analytics. This fragmentation hinders the ability to gain comprehensive insights and slows decision-making processes.
Costs
The rising demand for analytics, coupled with increasing sizes of data silos, leads to greater ETL, data copies, and movement costs. Consumption-based pricing models can create a "curiosity tax," making it expensive for organizations to freely explore and analyze their data.
Self-Service Barriers
Users often face delays in accessing data, relying on central teams for preparation and curation. This dependency restricts self-service capabilities, leading to inefficiencies and slower time-to-insight.
Lock-in
Proprietary and closed data ecosystems can create a risk of vendor lock-in. This limits the flexibility to use the best tools for specific tasks and hinders the ability to adapt to changing business needs.
Snowflake has embraced Apache Iceberg and open formats to address many of these issues. This allows organizations to bring in other platforms to smooth out these edges using a single copy of their data. Dremio takes this even further by connecting directly to your Snowflake account and working with your Snowflake and Iceberg tables. Additionally, Dremio can connect to Apache Iceberg catalogs like Nessie, Polaris, AWS Glue, and others. This capability allows Dremio to smooth frictions with a semantic layer, data unification, and more.
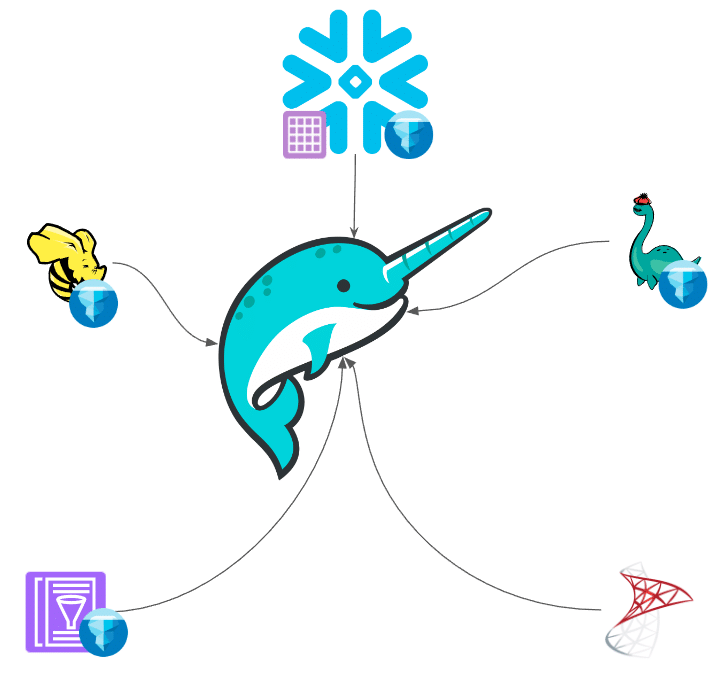
The Dremio Advantage
While Snowflake provides a robust foundation for data warehousing, integrating it with the Dremio Lakehouse Platform can significantly enhance its capabilities. Dremio addresses key friction points such as data silos, high costs, self-service barriers, and the risk of vendor lock-in. By leveraging Dremio's unique features, organizations can unify their data, optimize costs, empower users with self-service tools, and ensure a flexible, future-proof data architecture. Let's explore how Dremio tackles these challenges and adds value to your data ecosystem.
Siloed Data
Dremio addresses the challenge of siloed data by providing a unified data access layer that can connect to multiple data sources, including Snowflake, Apache Iceberg catalogs (Nessie, Polaris, AWS Glue), and more (include data on-prem to enable a hybrid data lakehouse). This unification provides organizations:
- Seamless Data Integration: Integrate data from various platforms without the need for complex and expensive ETL pipelines.
- Comprehensive Analytics: Perform cross-silo analytics effortlessly, enabling a holistic view of the data landscape.
- Enhanced Data Discovery: Easily discover and analyze data from disparate sources through a single platform.
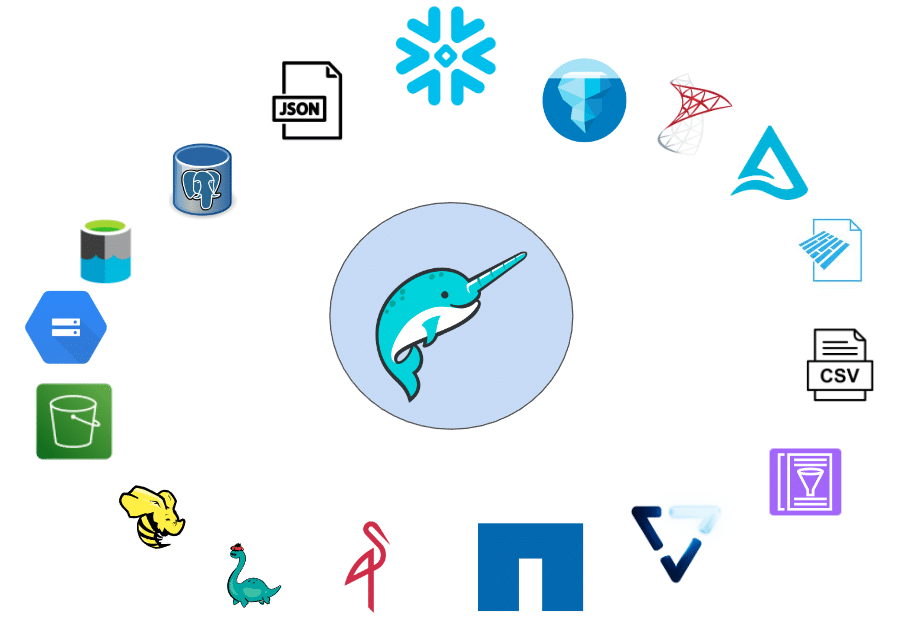
Costs
Dremio optimizes analytics costs by leveraging its intelligent query acceleration and semantic layer capabilities:
- Intelligent Query Acceleration: Accelerates queries by optimizing how data is accessed and processed, reducing the compute costs associated with large and complex queries.
- Cost Efficiency: Dremio helps organizations save on storage and processing costs by minimizing data movement and eliminating the need for multiple data copies.
- Predictable Budgeting: Provides predictable and manageable costs, allowing organizations to avoid the "curiosity tax" imposed by consumption-based pricing models.
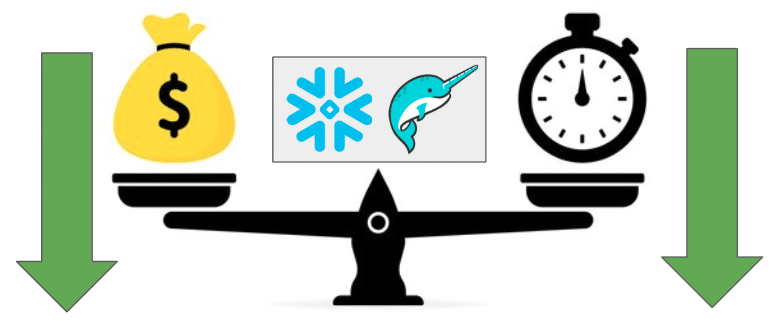
Self-Service Barriers
Dremio empowers users with self-service analytics, reducing dependency on central data teams and enabling faster time-to-insight:
- Intuitive Self-Service Tools: Provides user-friendly tools allowing data analysts and business users to access, prepare, and analyze data without extensive technical skills.
- Empowered Data Teams: Frees up central data teams to focus on more strategic tasks by reducing the bottleneck created by data preparation and curation requests.
- Accelerated Decision-Making: This feature enables users to quickly access the needed data, accelerating decision-making processes and fostering a data-driven culture.
Lock-in
Dremio's support for open standards and compatibility with Apache Iceberg ensures flexibility and reduces the risk of vendor lock-in:
- Open Data Formats: Embraces open data formats like Apache Iceberg, allowing organizations to future-proof their data architecture and avoid being tied to a single vendor.
- Interoperability: Ensures seamless interoperability with various data sources and tools, giving organizations the freedom to choose the best tools for their needs.
- Future-Proof Architecture: Builds on open standards, providing a flexible and adaptable data platform that can evolve with changing business requirements.
By integrating Dremio with your Snowflake data warehouse, you can overcome these challenges and create a more efficient, cost-effective, and flexible data platform. In the next section, we'll delve deeper into the specific benefits that Dremio brings to your data ecosystem.
Conclusion
Integrating Snowflake with the Dremio Lakehouse Platform offers a powerful combination that addresses some of the most pressing challenges in data management today. By unifying siloed data, optimizing analytics costs, enabling self-service capabilities, and avoiding vendor lock-in, Dremio complements and extends the value of your Snowflake data warehouse. This integrated approach allows organizations to harness the full potential of their data, driving faster, more informed decision-making and fostering a truly data-driven culture. As data grows in volume and complexity, leveraging Snowflake's and Dremio's strengths will ensure your data platform remains agile, efficient, and ready to meet future demands.
Let's discuss how to take your Snowflake Warehouse to the Next Level with Dremio! Meet with Us.
Here are Some Exercises for you to See Dremio’s Features at Work on Your Laptop
- Intro to Dremio, Nessie, and Apache Iceberg on Your Laptop
- From SQLServer -> Apache Iceberg -> BI Dashboard
- From MongoDB -> Apache Iceberg -> BI Dashboard
- From Postgres -> Apache Iceberg -> BI Dashboard
- From MySQL -> Apache Iceberg -> BI Dashboard
- From Elasticsearch -> Apache Iceberg -> BI Dashboard
- From Kafka -> Apache Iceberg -> Dremio